Faming Liang
Distinguished
Professor, Department of Statistics, Purdue
University, West Lafayette, IN 47907.
Office:
Math Building 500; Phone: (765)
494-4452; Email: fmliang@purdue.edu
Lecturing Assignment for 2024:
Editorial Service
-
Co-Editor,
Journal of Computational and Graphical Statistics,
2022-2025
-
Associate
Editor,
Journal of Computational and Graphical Statistics,
2006-2021
- Associate Editor, Annals of Mathematical
Sciences and Applications, 2015-present
-
Associate
Editor, Journal of American Statistical Association,
2010-2018
-
Associate Editor, Bayesian Analysis, 2010-2016
- Associate Editor, Technometrics,
2013-2017
-
Associate
editor, Biometrics, 2006-2008
Honors
-
IMS fellow, 2013.
-
ASA fellow, 2011.
-
Elected member, International Statistical Institute
(ISI), 2005.
-
Dean's Citation Award 2016.
- Youden Prize 2017
Research Interests:
-
Markov
Chain Monte Carlo
- Big Data
-
Bioinformatics
- Spatial Statistics
-
Machine
Learning
- Statistical Genetics
-
Stochastic
Optimization
Selected Publications:
-
Kendall,
W.S.,
Liang, F., and Wang, J.S. (2005) (Editors) Markov
Chain Monte Carlo: Innovations and Applications.
World Scientific: Singapore. ISBN 981-256-427-6.
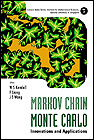
2. Liang, F.,
Liu, C. and Carroll, R.J. (2010) Advanced Markov chain Monte Carlo: Learning from
Past Samples. Wiley. ISBN:
978-0-470-74826-8.
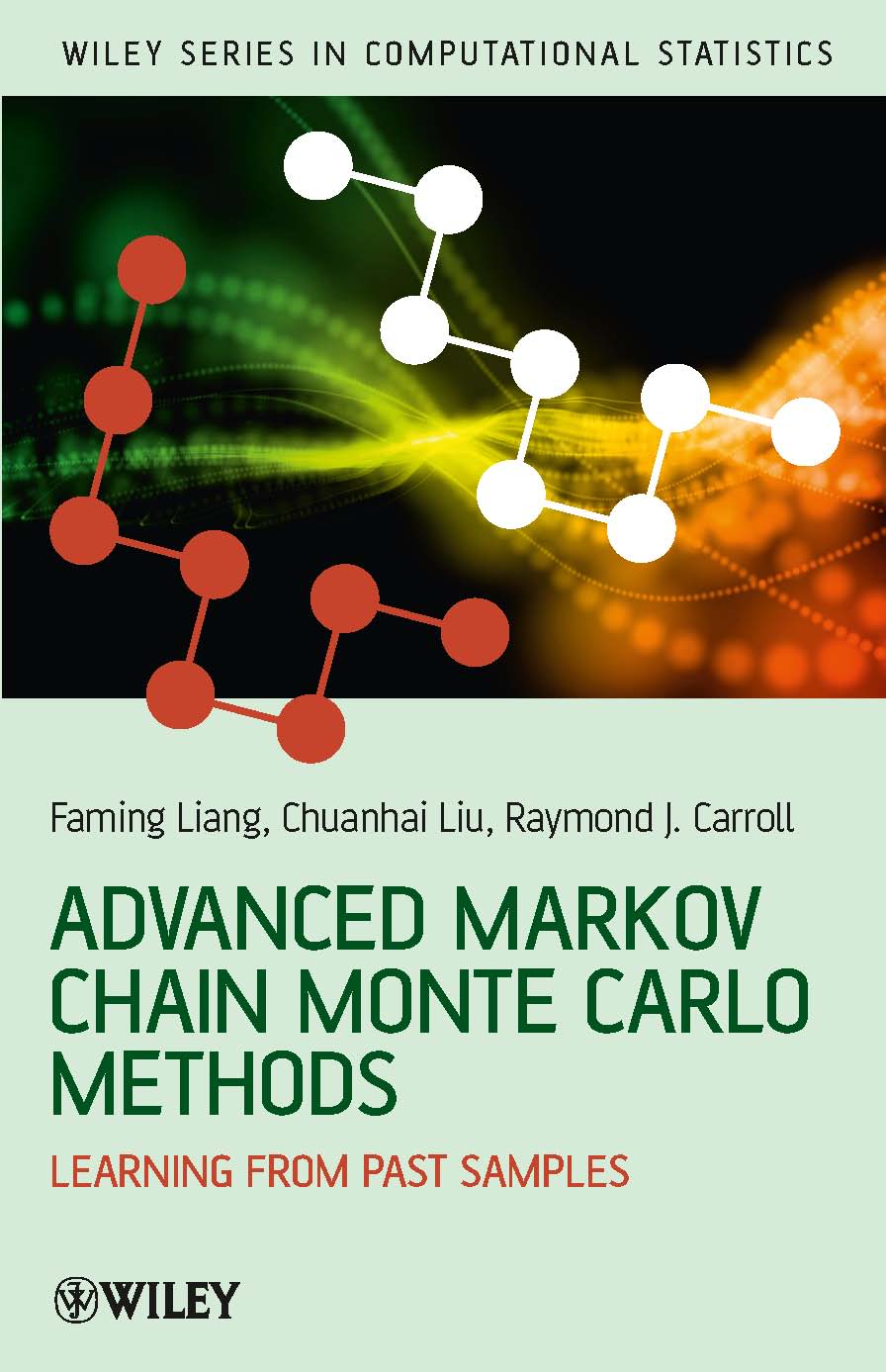
3. Liang, F. and Jia, B. (2023) Sparse
Graphical Modeling for High Dimensional Data: A
Paradigm of Conditional Independence Tests.
Chapman and Hall/CRC.
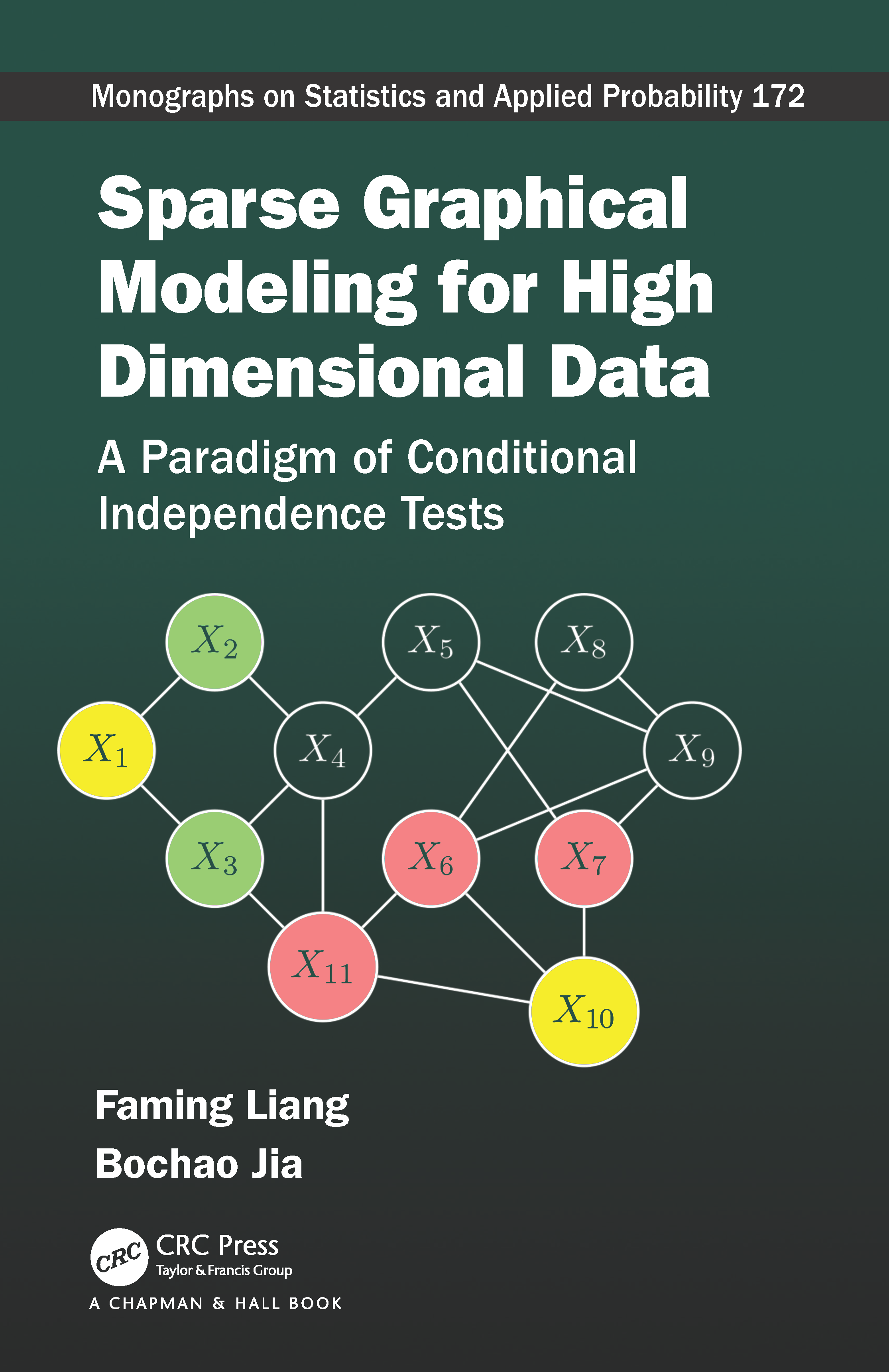
This book offers a comprehensive
framework for mastering the complexities of learning
high-dimensional sparse graphical models through the use
of conditional independent tests. These tests are
strategically conducted within a Markov neighborhood,
ensuring both the low dimensionality of the conditioning
set and their equivalence to the original
high-dimensional conditional independence tests. Key
highlights of the book include:
- Diverse Data Types: The
book provides in-depth treatments for various data
types, including Gaussian, Poisson, multinomial, and
mixed data, and addresses the challenges of missing
data, heterogeneous data, and data observed under
distinct conditions, making it a versatile resource
for a wide range of applications.
- Unified Approaches: The book
introduces unified strategies for covariate
adjustments, data integration, and network comparison,
streamlining the high-dimensional graphical modeling
process within complex data contexts.
- High-Dimensional Variable Selection:
The book delves into effective
high-dimensional variable selection, utilizing the
dependencies within covariates to aid researchers in
identifying the most pertinent features.
- High-Dimensional Inference:
The book equips readers with tools for
high-dimensional inference with the use of graphical
models formed by covariates. These methods
decompose the high-dimensional inference problem
into a series of low-dimensional ones, allowing
for the use of traditional tests, such as the
student-t test, in the high-dimensional context.
One notable feature of the methods
outlined in this book is their inherent parallel
structure for performing conditional independence tests.
This enables significant computational acceleration when
executed on a multi-core computer or a parallel
architecture. This book is intended for researchers,
scientists, and graduate students in various data
science disciplines. R code is available for numerical
examples in the book.
-
Cheng, Y. and Liang, F. (2016) RSAgeo:
Resampling-Based Analysis of Geostatistical Data,
available at https://cran.r-project.org/web/packages/RSAgeo/.
- Jia, B., Liang, F., Shi, R., and Xu, S.
(2017) equSA (Package and Manual):
Estimate Directed and Undirected Graphical Models and
Construct Networks.
-
Jia, B. and Liang, F. (2017) ICmiss Package and Manual.
-
Sun, Y., Song, Q. and Liang, F. (2021) Sparse Deep
Learning, available at https://github.com/sylydya/Consistent-Sparse-Deep-Learning-Theory-and-Computation
.
-
Wong,
W.H. and Liang, F. (1997) Dynamic
weighting in Monte Carlo and optimization, Proc.
Natl. Acad. Sci. USA, 94, 14220-14224.
-
Liang, F.
and Wong, W.H. (1999) Dynamic
weighting in simulations of spin systems, Phys.
Lett. A, 252, 257-262.
-
Cong, J.,
Kong, T., Xu, D., Liang, F., Liu, J.S., and Wong, W.H.
(1999) Relaxed
simulated tempering for VLSI floorplan designs,
Proc. Asia and South Pacific Design Automation
Conf., Hong Kong, pp13-16.
-
Cong, J.,
Kong, T., Xu, D., Liang, F., Liu, J.S., and Wong, W.H.
(2000) Dynamic
weighting Monte Carlo for constrained floorplan
design in mixed signal application,Proc. Asia
and South Pacific Design Automation Conf.,
Japan.
-
Sanderson
P, Taylor D., Ali M., Liew S.C., Couturier S., Lee G.,
Truong Y., Liang F., Gin K. and Holden H. (1999)
Development of a methodology for monitoring variations
in turbid waters draining modified wetlands in
southeast Sumatra, Indonesia: preliminary results for
suspended sediments, Eighth International
Symposium on the Interactions Between Sediments and
Water}, Beijing, pp. 13-17.
-
Liu,
J.S., Liang, F., and Wong. W.H. (2000) The
use of multiple-try method and local optimization in
Metropolis sampling, J. Amer. Statist.
Assoc.,95, 121-134.
-
Liang, F.
and Wong. W.H. (2000)
Evolutionary Monte Carlo sampling: applications to
$C_p$ model sampling and change-point problem. Statistica
Sinica,10, 317-342.
-
Truong,
Y. K., Liang, F., Sanderson, P. G., Taylor, D. and
Liew, S. C. (2000) M onitoring variations in turbid
waters draining modified wetlands in southeast Su
matra, Indonesia: A functional data analytic approach.
In Nonparametric approach to Knowledge Discovery,
Nara, Japan, December 14-17, 2000. Proceedings.
-
Liu,
J.S., Liang, F., and Wong. W.H. (2001) A
theory for dynamic weighting in Monte Carlo, J.
Amer. Statist. Assoc., 96, 561-573.
-
Liang, F.
and Wong. W.H. (2001) Real-parameter
evolutionary sampling with applications in Bayesian
Mixture Models, J. Amer. Statist. Assoc.,
96, 653-666.
-
Liang,
F., Truong, Y.K. and Wong, W.H. (2001) Automatic
Bayesian model averaging for linear regression and
applications in Bayesian curve fitting. Statistica
Sinica , 11, 1005-1029.
-
Liang, F.
and Wong, W.H. (2001) Evolutionary
Monte Carlo for Protein Folding simulations, Journal
of Chemical Physics , 115, 3374-3380.
-
Liang, F.
(2002) Some
connections between Bayesian and non-Bayesian
methods for regression model selection.
Statistics & Probability Letters , 57,
53-63.
-
Liang, F.
(2002) Dynamically
Weighted Importance Sampling in Monte Carlo
Computation, J. Amer. Statist. Assoc. ,
97, 807-821.
-
Liang, F.
(2003) An
Effective Bayesian Neural Network Classifier with a
Comparison Study to Support Vector Machine,
Neural Computation, 15,
1959-1989.
-
Liang,
F.
(2003) Use
of sequential structure in simulation from high
dimensional systems, Physical Review E,
67, 56101-56107.
-
Zhang,
J., Liang, F., Dassen, W. and de Gunst, M. (2003)
Search
for Haplotype-Interactions that are susceptible to
type I diabetes using unphased genotype data,
American J. Human Genetics, 73,
1385-1401.
-
Liang, F.
(2004). Generalized
1/k-Ensemble Algorithm, Physical Review E,
69, 66701-66707.
-
Liang,
F.
(2004) Annealing
Contour Monte Carlo for Structure Optimization in an
Off-lattice Protein Model. Journal of
Chemical Physics, 120, 6756-6763. (This
paper is selected by editors expert for
re-publication in the April 1, 2004 issue of Virtual
Journal of Biological Physcis Research.)
-
Liang,
F.
(2004) Annealing
contour Monte Carlo for neural network training.
Proceedings on Cybernetics and Informatics
Technologies, Systems and Applications, Volume III,
pp.130-135.
-
Liang, F.
and Kuk, Y.C.A. (2004) A
finite population estimation study with Bayesian
neural networks. Survey Methodology,
30, 219-234.
-
Liang, F.
(2005) Bayesian
neural networks for non-linear time series
forecasting. Statistics and Computing,
15, 13-29.
-
Liang,
F.
and Liu, C. (2005) Efficient
MCMC estimation of discrete distributions.
Computational Statistics and Data Analysis,
49, 1039-1052.
-
Liang, F.
(2005) Evidence
Evaluation for Bayesian Neural Networks. Neural
Computation, 17, 1385-1410.
-
Liang, F.
(2005) Generalized
Wang-Landau algorithm for Monte Carlo Computation.
J. Amer. Statist. Assoc., 100,
1311-1327.
-
Liang, F.
(2005) Determination
of normalizing constants for simulated tempering.
Physica A, 356, 468-480.
-
Liang, F.
(2005). Annotated bibliography: Advanced Markov chain
Monte Carlo methods. ISBA Bulletin,
12(4), 2-5.
-
Liang, F.
and Huang, J. (2006) Book Review: Statistical
and Computational Inverse Problems. Technometrics,
48, 146.
-
Liang, F.
(2006) A
theory on flat histogram Monte Carlo algorothms.
Journal of Statistical Physics, 122,
511-529.
-
Zhu, H.,
Liang, F., Gu, M. and Peterson, B. (2006)
Stochastic Approximation algorithms for estimation
of spatial mixed models. In Handbook
of Computing and Statistics with Applications,
Vol. 1 (eds. S.Y. Lee),
Elsevier. pp.399-421.
-
Liang,
F., Liu, C. and Wang, N. (2007) A
sequentail Bayesian procedure for
identification of differentially expressed genes.
Statistica Sinica, 12, 571-597.
-
Liang,
F., Liu, C. and Carroll, R.J. (2007) Stochastic
Approximation in Monte Carlo Computation.
J. Amer. Statist. Assoc., 102, 305-320.
-
Liang, F.
and Wang, N. (2007) Dynamic
Hierarchical Clustering of Gene Expression Profiles.
Pattern Recognition Letters, 28,
1062-1076.
-
Liang, F.
(2007) Use
of SVD-based probit transformation in clustering
gene expression profiles. Computational
Statistics and Data Analysis, 51,
6355-6366.
-
Liang, F.
(2007) Continuous
Contour Monte Carlo for Marginal Density Estimation
with an Application
to a Spatial Statistical Model, Journal of
Computational and Graphical Statistics, 16(3),
608-632.
-
Liang,
F. (2007) Annealing
Stochastic Approximation Monte Carlo for Neural
Network Training. Machine Learning,
68(3), 201-233.
-
Cheon,
S.
and Liang, F. (2008) Phylogenetic
Tree Reconstruction Using Sequential Stochastic
Approximation Monte Carlo. BioSystems,
91, 94-107.
-
Liang, F.
(2008) Stochastic
Approximation Monte Carlo for MLP Learning.
In Encyclopedia of Artificial Intelligence,
(eds. J.R.R. Dopico, J.D. de la Calle, and A.P.
Sierra), pp.1482-1489.
-
Zhang,
J., J Rggieli, M. Schipper, M. Entius, F. Liang, J.
Koerselman, H Ruven, Y van der Graaf, D. Grobbee, and
P. Doevendans (2008) Inflammatory
gene haplotype-interaction networks involved in
coronary collateral formation.
Human Heredity, 66, 252-264.
-
Liang, F.
and Zhang, J. (2008) Estimating
FDR under general dependence using stochastic
approximation. Biometrika, 95(4),
961-977.
-
Liang,
F.
(2008) Clustering
gene expression profiles using mixture model
ensemble averaging approach. JP
Journal of Biostatistics, 2(1), 57-80.
-
Zhang, J.
and Liang, F. (2008) Convergence
of stochastic approximation under irregular
conditions. StatisticaNeerlandica, 62,
393-403.
-
Yuan, R.,
Ding, Y., and Liang, F. (2008) Adaptive
Evolutionary Monte Carlo for Optimizations with
Applications to Sensor Placement Problems.
Statistics and Computing, 18, 375-390.
-
Liang, F.
(2009)
Improving SAMC Using Smoothing Methods: Theory and
Applications to Bayesian Model Selection Problems.
The Annals of Statistics, 37,
2626-2654.
-
Liang, F.
(2009) On
the use of SAMC for Monte Carlo integration. Statistics
& Probability Letters, 79, 581-587.
-
Liang,
F.
and Zhang, J. (2009) Learning
Bayesian Networks for Discrete Data. Computational
Statistics & Data Analysis, 53,
865-876.
-
Zhang,
X.S., Liang, F., Srinivasan, R., and Van Liew, M.
(2009)
Estimating uncertainty of streamflow simulation
using Bayesian neural networks. Water
Resources Research, 45, W02403.
-
Cheon,
S.
and Liang, F. (2009) Bayesian
phylogeny analysis via stochastic approximation
Monte Carlo. Molecular Phylogenetic
& Evolution, 53, 394-403.
-
Wu, M.,
Liang, F. and Tian, Y. (2009) Bayesian
Modeling of ChIP-chip Data Using Latent Variables. BMCBioinformatics,
10: 352.
-
Xie, Y., Zhang, Y., and Liang, F.
(2009) Crash injury severity analysis using Bayesian
ordered probit models. Journal of
Transportation Engineering, 135(1),
18-25.
-
Liang,
F.
(2009) Learning Bayesian Networks for Gene Expression
Data. In Bayesian Modeling in Bioinformatics
(Eds. D.K. Dey, S. Ghosh and B.K. Mallick),
pp.349-367.
- Liang, F. and Cheon, S. (2009) Monte Carlo dynamically
weighted importance sampling for spatial models with
intractable normalizing constants. Journal of Physics:
Conference Series, 197, 012004.
-
Zhang,
P., Hill, C., Xia, Y., and Liang, F. (2010)
Modeling the relationship between EDI implementation
and firm performance improvement with neural
networks. IEEE Transactions on Automation
Science and Engineering, 7, 96-110.
-
Liang, F.
(2010) A double Metropolis-Hastings sampler for
spatial models with intractable normalizing constants. Journal of Statistical
Computing and Simulation, 80, 1007-1022.
-
Martinez,
J.N., Liang, F., Zhou, L., and Carroll, R.J. (2010)
Longitudinal Functional Principal Component
Modeling via Stochastic Approximation Monte Carlo. Canadian
Journal of Statistics, 38, 256-270.
-
Mo, Q.
and Liang, F. (2010) Bayesian modeling of
ChIP-chip data through a high-order Ising model.
Biometrics, 66,
1284-1294.
-
Zhang, J.
and Liang, F. (2010) Exponential power mixture models
for clustering. Biometrics, 66, 1078-1086.
-
Mo, Q.
and Liang, F. (2010) A hidden Ising model for
ChIP-chip data analysis. Bioinformatics, 26, 777-783.
-
Liang, F.
(2010) Trajectory averaging for stochastic
approximation MCMC algorithms. Annals of
Statistics, 38,
2823-2856.
-
Wu, M.
and Liang, F. (2010) Testing Multuiple
Hypotheses Using Population Information of
Samples. JPJournal of Biostatistics, 4, 181-201.
-
Liang, F.
(2011) Evolutionary Stochastic Approximation Monte
Carlo for Global Optimization. Statistics
and Computing,
21, 375-393.
-
Yin, G., Ma, Y., Liang, F. and Yuan, Y. (2011)
Stochastic generalized method of moments. Journal of Computational
and Graphical Statistics, 20, 714-727.
-
Yu, K., Liang, F., Chatterjee, N., and Ciampa, J.
(2011) Efficient p-Value Evaluation for
Resampling-based tests. Biostatistics, 12, 582-593.
-
Zhang, N., Li, X., Tao k., Jiang, L., Ma, T.,
Yan, S., Yuan, C. Moran, M.S., Liang, F., Haffty, B.G.
and Yang, Q. (2011) BCL-2 (-938C>A) polymorphism is
associated with breast cancer susceptibility. BMC Medical Genetics,
12:48.
-
Cheon, S. and Liang, F. (2011) Folding small
proteins via annealing stochastic approximatio Monte
Carlo. BioSystems,
105, 243-249.
-
Zhang, X., Liang, F., Yu, B. and Zong, Z. (2011)
Explicitly integrating parameter, input and
structure uncertainties into Bayesian neural networks
for probabilistic hydrologic forecasting. Journal of Hydrology,
409, 696-709.
-
Park, J. and Liang, F. (2012) Bayesian analysis of
geostatistical models with an auxiliary lattice. Journal of computational
and Graphical Statistics, 21, 453-475.
-
Shi, X., Zhu, H., Ibrahim, J.G., Liang, F.,
Lieberman, J. and Styner, M. (2012) Intrinsic
regression models for medial representation of
subcortical structures. J. Amer. Statist. Assoc., 107, 12-23.
-
Yu, K., Wacholder, S., Wheeler, W., Wang, Z.,
Caporaso, N., Landi, M.T., Liang, F. (2012) A
flexible Bayesian model for studying gene-environment
interaction.
PLoS Genetics, 8(1): e1002482.
-
Jin, I.K. and Liang, F. (2013) Fitting social network
models using varying truncation stochastic
approximation MCMC algorithm. Journal of Computational
and Graphical Statistics, 22,
927-952.
-
Jin, I.K. and Liang, F. (2013) Bayesian SAMC for
distributions with intractable normalizing
constants, Computational
Statistics & Data Analysis, in press.
-
Ryu, D., Liang, F. and Mallick, B.K. (2013). Sea Surface
Temperature Modeling using Radial Basis Function
Networks with a Dynamically Weighted Particle
Filter. J.
Amer. Statist. Assoc., 108, 111-123.
-
Liang, F., Cheng, Y., Song, Q., Park, J., and Yang,
P. (2013). A Resampling-based Stochastic Approximation
Method for Analysis of Large Geostatistical Data. J. Amer. Statist.
Assoc., 108, 325-339.
-
Zhou, C., Yang, P., Dessler, A.E., and Liang, F.
(2013). Statistic of horizontally oriented ice
cloud crystals in optically thick clouds. IEEE Geoscience and
Remote Sensing Letters, 10, 986-990.
-
Liang, F., Song, Q., and Yu, K. (2013).
Bayesian Subset Modeling for High Dimensional
Generalized Linear Models. J. Amer. Statist. Assoc.,
108, 589-606.
-
Liang, F. and Xiong, M. (2013). Bayesian
detection of disease-associated rare variants under
posterior consistency. PLoS One, 8(7),
e69633.
- Pourhabib, A., Liang, F. and Ding, Y. (2013). Bayesian
site selection for fast Gaussian process regression. IIE
Transactions, in press.
-
Liang, F. and Jin, I.K. (2013). A Monte Carlo
Metropolis-Hastings Algorithm for Sampling from
Distributions with Intractable Normalizing Constants.
Neural Computation, 25, 2199-2234.
-
Cheon, S., Liang, F., Chen, Y., and Yu, K.
(2014). Stochastic Approximation Monte Carlo
Importance Sampling for Approximating Exact
Conditional Probabilities. Statistics and
Computing, 24, 505-520.
-
Pourhabib, A., Liang, F. and Ding, Y. (2014).
Bayesian site selection for fast Gaussian process
regression. IIE Transactions, 46, 543-555.
-
Zhang, H., Shi, J., Liang, F., Wheeler, W.,
Stolzenberg-Solomon R., and Yu K. (2014). A fast
multilocus test with adaptive SNP selection for
large-scale genetic-association studies. European
Journal of Human Genetics, 22, 696-702.
-
Cheng, Y., Gao, X., and Liang, F. (2014). Bayesian
Peak Picking for NMR Spectra. Genomics, Proteomics
& Bioinformatics, 12, 39-47.
- Jin, I.K. and Liang, F. (2014) Bayesian SAMC for
distributions with intractable normalizing
constants, Computational
Statistics & Data Analysis, 71, 402-416.
-
Song, Q., Wu, M. and Liang, F. (2014). Weak
Convergence Rates of Population versus Single-Chain
Stochastic Approximation MCMC Algorithms. Advances
in Applied Probability, 46, 1059-1083. (Supplementary
Material)
-
Liang, F., Cheng, Y., and Guang, L. (2014). Simulated
Stochastic Approximation Annealing for Global
Optimization with a Square-root Cooling Schedule.
J. Amer. Statist. Assoc., 109, 847-863.
-
Liang, F. (2014). An Overview of Stochastic
Approximation Monte Carlo. WIREs Computational
Statistics, 6,
240-254.
-
Xu, G., Liang, F. and Genton, M. (2015). A Bayesian
Spatio-Temporal Geostatistical Model with an Auxiliary
Lattice for Large Datasets. Statistica Sinica,
25,61-79.
- Cheng, Y. and Liang, F. (2015) Discussion on "Modeling
an Augmented Lagrangian for Improved Blackbox
Constrained Optimization" by Gramacy et al. Technometrics, 61, 39.
-
Song, Q. and Liang, F. (2015). A Split-and-Merge
Bayesian Variable Selection Approach for Ultra-high
dimensional Regression. J. Royal Statist. Soc. B, 77(5), 947-972.
-
Song, Q. and Liang, F. (2015). High Dimensional
Variable Selection with Reciprocal L1-Regularization.
J. Amer. Statist. Assoc., 110, 1607-1620.
- Liang, F., Song, Q. and Qiu, P. (2015). An Equivalent
Measure of Partial Correlation Coefficients for High
Dimensional Gaussian Graphical Models. J. Amer.
Statist. Assoc., 110,
1248-1265.
-
Liang, F., Jin, I.H., Song, Q. and Liu, J.S. (2016).
An Adaptive Exchange Algorithm for Sampling from
Distribution with Intractable Normalizing Constants. J.
Amer. Statist. Assoc., 111, 377-393.
-
Liang, F., Shi, R. and Mo, Q. (2016). A
split-and-merge approach for singular value
decomposition of large-scale matrices. Statistics and Its
Interface, 9, 453-459.
-
Liang, F., Kim, J. and Song, Q. (2016). A
Bootstrap Metropolis-Hastings Algorithm for Bayesian
Analysis of Big Data. Technometrics, 58, 304-318.
- Lamba, V., Jia, B. and Liang, F. (2016). STAT5A and
STAT5B have opposite correlations with drug response
gene expression. Biochemical
and Biophysical Research Communications,
479(2): 117-124.
-
Georgios Karagiannis, Bledar A. Konomi, Guang
Lin, and Faming Liang (2017). Parallel and Interactive
Stochastic Approximation Annealing Algorithms for
Global Optimization. Statistics and Computing, 27(4),
927-945.
- Tianzhou Ma, Faming Liang, and George C.
Tseng (2017). Biomarker detection and categorization in
ribonucleic acid sequencing meta-analysis using Bayesian
hierarchical models. Applied
Statistics, 66(4), 847-867.
-
Luo, X., Huang, J., Liang, F., Canalesm J.R.,
Wistuba, I.I., Gazdar, A., Xie, Y., and Xiao, G.
(2017). Comprehensive Computational Pathological Image
Analyses Predict Lung Cancer Prognosis. Journal of Thoracic
Oncology, 12(3), 501-509.
-
Lee, S., Liang, F., Cai, L. and Xiao, G. (2017)
Integrative analysis of gene networks and their
application to lung adenocarinoma studies. Cancer Informatics:
16.
-
Li, X., Campbell-Thompson, M., Wasserfall, C.H.,
McGrail, K., Posgai, A., Schultz, A.R., Brusko, T.M.,
Shuster, J., Liang, F., Muir, A., Schatz, D., Haller,
M. and Atkinson, M.A. (2017). Serum tryosinogen
levels in pre-type 1 diabetes--A novel biomarker
associating with disease risk. Diabetes Care,
40(4), 577-582.
-
Jia, B., Xu, S., Lamba, V., Xiao, G., and
Liang, F. (2017) Inference of Genetic Network with
Next Generation Sequencing Data. Biometrics,
73(4), 1221-1230.
- Sundaresan, V., Lin, V.T., Liang, F., Kaye, F.J., and
Zhou, L. (2017). Significantly mutated genes and
regulatory pathways in SCLC--A Meta Analysis. Cancer
Genetics, 216-217, 20-28.
-
Yu, D., Lim, J., Wang, X., Tang, H., Liang, F. and
Xiao, G. (2017) Enhanced construction of gene
regulatory networks using hub gene information. BMC Bioinformatics,
18(1):186.
-
Li, Qi, Yi, F., Wang, T., Xiao, G. and Liang, F.
(2017) Lung cancer pathological image analysis using a
hidden Potts model. Cancer
Informatics, 16, 1-9. Algorithm
Implementation
-
Ma, T., Liang, F., Oesterreich, S., and Tseng, G.C.
(2017). A joint Bayesian modeling for integrating
microarray and RNA-seq transcriptomic data. Journal of Computational
Biology, 24(7), 647-662.
-
Xue, J. and Liang, F. (2017). A Robust Model-Free
Feature Screening Method for Ultrahigh-Dimensional
Data. Journal of
Computational and Graphical Statistics,
26(4), 803-813.
-
Lee, S., Liang, F., Cai, L. and Xiao, G. (2018) A
two-stage approach of gene network analysis for
high-dimensional heterogeneous data. Biostatistics,
19(2), 216-232 .
-
Ryu, D., Bilgili, D., Ergonul, O., Liang, F., and
Ebrahimi, N. (2018). A Bayesian generalized linear
model for Crimean-Congo hemorrhagic fever
incidents. Journal of Agricultural,
Biological, and Environmental Statistics, 23(1),
153-170.
-
Liang, F., Li, Q. and Zhou, L. (2018) Bayesian
Neural Networks for Selection of drug sensitive Genes.
J. Amer. Statist. Assoc., 113, 955-972.
-
Liang, F., Jia, B., Xue, J., Li, Q. and Luo, Y.
(2018) An imputation-regularized optimization
algorithm for high-dimensional missing data problems
and beyond. J. Royal Statist. Soc. B,
80(5), 899-926. (See arXiv:1802.02251 for an
early version of this paper).
- Zhang, A., Fang, J., Liang, F., Calhoun, V.D.,
and Wang, Y.-P. (2018). Aberrant Brain Connectivity in
Schizophrenia Detected via a Fast Gaussian Graphical
Model. IEEE Journal of Biomedical and Health
Informatics (J-BHI), PP:1. DOI:
10.1109/JBHI.2018.2854659.
-
Li, Q., Wang, X., Liang, F., Yi, F., Xie, Y.,
Gazdar, A., and Xiao, G. (2019). A Bayesian
Hidden Potts Mixture model for Analyzing Lung Cancer
Pathological Images. Biostatistics,
20(4):565-581.
-
Shi, R., Liang, F., Song, Q., Luo, Y., and Ghosh, M.
(2019). A Blockwise Consistency Method for Parameter
Estimation of Complex Models. Sankhya B, in
press, https://doi.org/10.1007/s13571-018-0183-0.
-
Li, Q., Shi, R. and Liang, F. (2019). Drug
Sensitivity Prediction with High-Dimensional Mixture
Regression (Algorithm
Implementation). PLoS One, 14(2):
e0212108.
- Wu, M., Luo, Y. and Liang, F. (2019) Accelerate
training of restricted Boltzmann machine via iterative
conditional maximum likelihood estimation. Statistics
and Its Interface, 12(3):377-385.
-
Xue, J. and Liang, F. (2019) Double-parallel
Monte Carlo for Bayesian analysis of big data.
Statistics and Computing, 29(1), 23-32.
-
Xu, S., Jia, B. and Liang, F. (2019). Learning
moral graphs in construction of high-dimensional
Bayesian Networks for mixed data. Neural
Computation, 31(6), 1183-1214.
-
Qiwei Li, Xinlei Wang, Faming Liang and
Guanghua Xiao. (2019). A Bayesian mark interaction
model for analysis of tumor pathology images. Annals
of Applied Statistics, 13(3),
1708-1734.
-
Mingqi Wu, Yinsen Miao, Neilkunal Panchal, Daniel
Kowal, Marina Vannucci, Jeremy Vila, and Faming
Liang (2019). Stochastic Clustering and Pattern
Matching for Real Time Geosteering. Geophysics,
84(5), ID13-ID24.
-
Aiying Zhang, Biao Cai, Wenxing Hu, Bochao
Jia, Faming Liang, Tony W. Wilson, Julia M.
Stephen, Vince D. Calhoun, Yu-Ping Wang (2019).
Joint Bayesian-Incorporating Estimation of Multiple
Gaussian Graphical Models to Study Brain
Connectivity Development in Adolescence. IEEE
Transactions on Medical Imaging, 39(2), 357-365.
-
Wei Deng, Xiao Zhang, Faming Liang, and Guang Lin
(2019). An Adaptive Empirical Bayesian Method for
Sparse Deep Learning. NeurIPS 2019,
published.
-
Song, Q., Sun, Y., Ye, M., and Liang, F. (2020).
Extended Stochastic Gradient MCMC for Large-Scale
Bayesian Variable Selection. Biometrika,
107(4), 997–1004.
-
Jia, B. and Liang, F. (2020). Joint Estimation
of Multiple Mixed Graphical Models for Pan-Cancer
Network Analysis. STAT, 9(1), e271.
-
Wei Deng, Qi Feng, Liyao Gao, Faming Liang, and
Guang Lin (2020). Non-convex Learning via Replica
Exchange Stochastic Gradient MCMC. ICML 2020.
-
Ick-Hoon Jin, Shin Minsuk, Jonghyun Yun, Faming
Liang (2020). Stochastic approximation Hamiltonian
Monte Carlo. Journal of Statistical
Computation and Simulation, 90(17), 3135-3156.
-
Deng, W., Lin, G. and Liang, F. (2020). A
Contour Stochastic Gradient Langevin Dynamics
Algorithm for Simulations of Multi-modal
Distributions. NeurIPS 2020.
-
Bochao Jia, Faming Liang, and TEDDY Study Group
(2021). Fast hybrid Bayesian integrative learning of
multiple gene regulatory networks for type 1
diabetes. Biostatistics, 22(2),
233–249.
-
Wei Deng, Qi Feng, Georgios Karagiannis, Guang
Lin, and Faming Liang (2021). Accelerating Convergence
of Replica Exchange Stochastic Gradient MCMC via
Variance Reduction. (arXiv:2010.01084) ICLR 2021.
-
Sun, Y., Song, Q., and Liang, F. (2021).
Consistent Sparse Deep Learning: Theory and
Computation. Journal of the American
Statistical Association, in press.
-
Fabrizio Cicala, Weicheng Wang, Tianhao
Wang, Ninghui Li, Elisa Bertino, Faming Liang,
Yang Yang (2021). PURE: A Framework for Analyzing
Proximity-based Contact Tracing Protocols. ACM
Computing Surveys, in press.
-
Liang, S., Huang, W.-H., and Liang, F. (2021).
Sufficient dimension reduction with deep neural
networks for phenotype prediction. Proceedings
of the 3rd International Conferences on
Statistics: Theory and Applications (ICSTA'21).
-
Sun, Y., Xiong, W. and Liang, F. (2021). Sparse deep
learning: A new framework immune to local traps and
miscalibration. NeurIPS 2021.
-
Liang, F., Xue, J. and Jia, B. (2022). Markov
neighborhood regression for high-dimensional
inference. Journal of the American Statistical
Association, 117(539), 1200-1214.
-
Kim, S., Song, Q., and Liang, F. (2022)
Stochastic Gradient Langevin Dynamics with Adaptive
Drifts. Journal of Statistical Computation
and Simulation, 92(2) 318-336.
-
Sun, Y., Song, Q., and Liang, F. (2022)
Learning sparse deep neural networks with a
spike-and-slab prior. Statistics and Probability
Letters, 180, 109246.
-
Sun, Y. and Liang, F. (2022). A kernel-expanded
stochastic neural network. Journal of the Royal
Statistical Society Series B, 84, 547-578.
-
Deng, W., Liang, S., Hao, B., Lin, G. and
Liang, F. (2022). Interacting Contour Stochastic
Gradient Langevin Dynamics. ICLR 2022.
-
Sun, L. and Liang, F. (2022) Markov Neighborhood
Regression for Statistical Inference of
High-Dimensional Generalized Linear Models. Statistics
in Medicine, 41, 4057-4078.
-
Deng, W., Lin, G. and Liang, F. (2022). An
Adaptively Weighted Stochastic Gradient MCMC
Algorithm for Global Optimization in Deep
Learning. Statistics and Computing, 32, 58.
- Liang, S., Sun, Y., and Liang, F. (2022). Nonlinear
sufficient dimension reduction with a stochastic neural
network. NeurIPS 2022.
-
Zhang, P., Dong, T., Li, N. and Liang, F. (2023).
Identification of Factors Impacting on the
Transmission and Mortality of COVID-19. Applied
Statistics (COVID-19 special issue), 50
(11-12), 2624-2627.
-
Song, Q. and Liang, F. (2023). Nearly optimal
Bayesian Shrinkage for high dimensional regression
(arXiv:1712.08964). China Science
Mathematics, 66(2), 409-442.
-
Dong, T., Zhang, P. and Liang, F. (2023). A
Stochastic Approximation-Langevinized Ensemble Kalman
Filter for State Space Models with Unknown Parameters.
Journal of Computational and
Graphical Statistics, 32(2), 448-469.
-
Liang, S. and Liang, F. (2023). A Double
Regression Method for Graphical Modeling of
High-dimensional Nonlinear and Non-Gaussian Data. Statistics
and Its Interface, in press.
- Deng, W., Zhang, Q., Feng, Q., Liang, F., and
Lin, G. (2023). Non-reversible Parallel Tempering for
posterior approximation in Deep Learning. AAAI 2023,
7332-7339.
-
Zhang, M., Sun, Y., and Liang, F. (2023).
Sparse Deep Learning for Time Series Data: Theory and
Applications. NeurIPS 2023.
-
Zhang, P., Song, Q., and Liang, F. (2024). A
Langevinized Ensemble Kalman Filter for Large-Scale
Dynamic Learning. Statistica Sinica, in press.
- Zhang, Q. and Liang, F. (2024). Bayesian analysis of
exponential random graph models using stochastic
gradient Markov chain Monte Carlo. Bayesian Analysis,
in press.
-
Kim, S., Song, Q., and Liang, F. (2024). A new
paradigm for generative adversarial networks based on
randomized decision rules. Statistica Sinica,
in press.
- Zhang, P., Dong, T. and Liang, F. (2024). An
Extended Langevinized Ensemble Kalman Filter for
non-Gaussian Dynamic Systems. Computational
Statistics, in press.
-
Shih, F. and Liang, F. (2024). Fast Value
Tracking for Deep Reinforcement Learning. ICLR 2024,
in press.
-
Yaxin Fang and Faming Liang (2024).
Causal-StoNet: Causal Inference for High-Dimensional
Complex Data. ICLR 2024, in press.
-
Sun, L., Zhang, A. and Liang, F. (2024). Time-varying
dynamic Bayesian network
learning for an fMRI Study of Emotion Processing. Statistics
in Medicine, in press.
|