Keynote Speaker
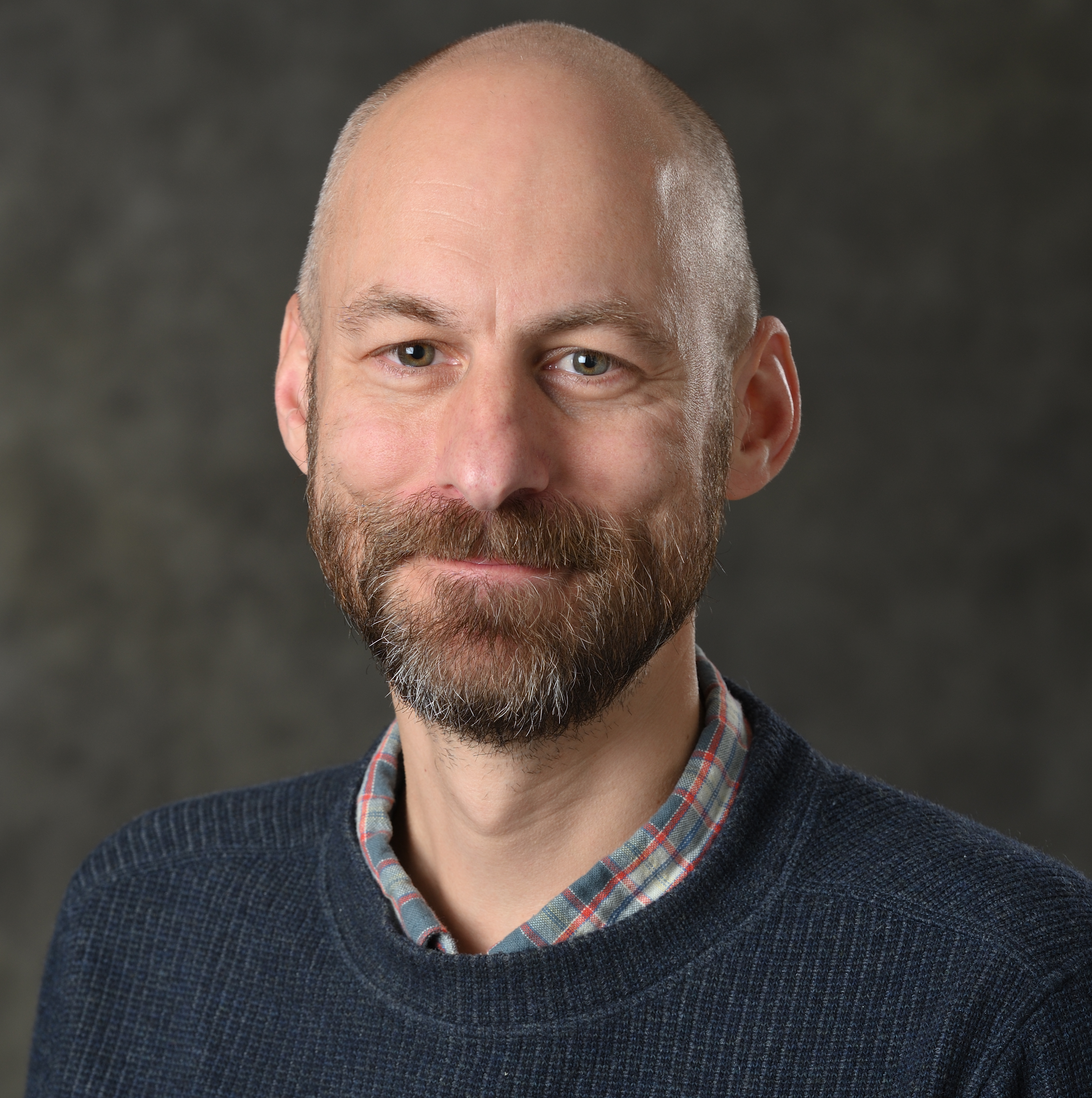
Andrew Finley is a Professor at Michigan State University with a joint appointment in the Department of Forestry and Department of Geography, Environment, and Spatial Sciences. He is also an adjunct Professor in the Department of Statistics and Probability and member of the interdisciplinary Ecology, Evolutionary Biology, and Behavior Graduate Program faculty. He earned his PhD in Forest Biometrics and MS in Statistics from the University of Minnesota (2006), MS in Forestry from the University of Massachusetts (2003), and BS in Forest Science from The Pennsylvania State University (2000).
His research advances methodologies and software for monitoring and modeling environmental processes, Bayesian statistics, spatial statistics, and statistical computing. A central theme in his research and teaching is the use of hierarchical models to integrate information from disparate sources to improve inference and prediction. He has authored a book, five R software packages, and more than 100 scientific publications in the areas of Bayesian and spatio-temporal statistics with applications in forestry, ecology, agriculture, and environmental science.
Keynote Talk: Tackling large spatial datasets via dimension reduction, induced sparsity, and distributed computing: A case study in forestry applications.
Spatial process models for analyzing geostatistical data entail computations that become prohibitive as the number of spatial locations increases. Developing statistical and computational methods to tackle this challenge of dimensionality is an active area of research. In practice, workable solutions commonly require a combination of complementary modeling and computing tools. This talk highlights a class of highly scalable Nearest Neighbor Gaussian Process (NNGP) models that provide fully model-based inference for large geostatistical datasets. Presentation of the NNGP is motivated by a study that aims to predict forest biomass at the species-level across the continental US. Here, both the number of locations and number of outcomes at each location is large. The proposed hierarchical model addresses these sources of dimensionality via a spatial latent factor model with NNGPs used as sparsity-inducing priors on each factor. Model parameters are estimated using a computationally efficient Markov chain Monte Carlo (MCMC) algorithm, for which scalability is achieved by avoiding storage or decomposition of large matrices and strategic use of shared memory parallelism.