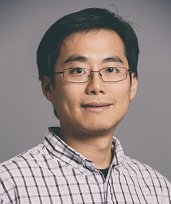
Off-policy Estimation in Reinforcement Learning: Algorithms and Applications
Lihong Li
Research Scientist
Google Inc.
Venue: LWSN 1142
Refreshments: 10:00 a.m. in HAAS 111
Abstract:
In many real-world applications of reinforcement learning (RL) such as healthcare, dialogue systems and robotics, running a new policy on humans or robots can be costly or risky. This gives rise to the critical need for off-policy estimation, that is, estimate the average reward of a target policy given data that was previously collected by another policy. In this talk, we will review some of the key techniques, such as inverse propensity score and doubly robust methods, as well as a few important applications. We will then describe a recent work that for the first time makes off-policy estimation practical for long- or even infinite-horizon RL problems.
Bio: Lihong Li is a research scientist at Google. He obtained a PhD degree in Computer Science from Rutgers University. After that, he has held research positions in Yahoo! Research and Microsoft Research. His main research interests are in reinforcement learning, including contextual bandits, and other related problems in AI. His work has found applications in recommendation, advertising, Web search and conversation systems, and has won best paper awards at ICML, AISTATS and WSDM. He serves as area chair or senior program committee member at major AI/ML conferences such as AAAI, ICLR, ICML, IJCAI and NIPS.