Congratulations May 2009 Graduates!
05-15-2009
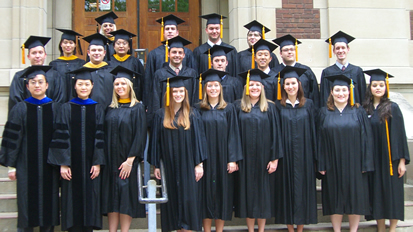
(click image to see the larger version)
A New Tradition: May 2009 Statistics and Actuarial Science graduates were invited to gather in front of Haas Hall before commencement for a group photo.
Pictured left to right
First Row: Zhongyin John Daye, Xinge Jessie Jeng, Megan Reineke, Rachel Smith, Kristen Dyson, Sarah Haynes, Katherine Quinn, Kathryn Patterson, Noor Al Sayed
Second Row: Samuel Crawford, Glen DePalma, Craig Allen, Jacob Dummitt, Andrew Chung, Myles Handley, Nathan Orlow
Third Row: Yuan Yang, Yan Sun, Michael Hoyer, Jonathan Schaab, Steven Balog
Top Row: Dina El Souda
The Department of Statistics would like to congratulate all of our May 2009 graduates. On May 15, 2009, Purdue University and the Department of Statistics awarded degrees to the following people:
Ph.D. Graduates (Advisor) Dissertation Title
- Mr. Zhongyin (John) Daye (Michael Zhu) "Some New Approaches in High-Dimensional Variable Selection and Regression"
- Ms. Xinge (Jessie) Jeng (Jayanta Ghosh, Jiashun Jin) "Covariance Adaptation and Regularization in Large-Scale Hypothesis Testing and High-Dimensional Regression"
- Mr. Nikita Tuzov (Frederi Viens) "Mutual Fund Performance and Evaluation Methodology and Local False Discovery Rate Approach"
- Ms. Lingmin Zeng (Jun Xie) "Group Variable Selection Methods and Their Applications in Analyses and Genomic Data"
M.S. Graduates
- Mr. Brian Denton
- Mr. Glen DePalma
- Ms. Dina El Souda
- Ms. Yen-Ning Huang
- Mr. Michael Lawlor
- Mr. Yongjin Ma
- Mr. Yanhui Mi
- Ms. Megan Reineke
- Mr. Gang Shen
- Ms. Yan Sun
- Ms. Yuan Yang
- Mr. Zuoyi Zhang
- Mr. Shaohua Zhou
Graduate Certificates
- Mr. Abhiskek Bhargava
- Ms. Huijie Wang
- Mr. Shih-Ching Wu
- Mr. Chao-Lung Yang
Undergraduates in Statistics
- Ms. Noor M. Al Sayed
- Mr. Craig A. Allen
- Mr. Steven M. Balog
- Mr. Andrew Chung
- Mr. Samuel T. Crawford
- Mr. Adam R. Crone
- Ms. Kristen E. Dyson
- Ms. Emily A. Haas
- Mr. Myles A. Handley
- Ms. Sarah M. Haynes
- Ms. Gita A. Himawan
- Mr. Daniel P. Holder
- Mr. Michael D. Hoyer
- Mr. Dhaval M. Jadhav
- Ms. Petrina Kusliawan
- Ms. Lisa McGuire
- Mr. Brett R. Newman
- Ms. Joy A. Nyenhuis-Rouch
- Mr. Samuel V. Oglesby
- Mr. Nathan N. Orlow
- Ms. Kathryn R. Patterson
- Ms. Katherine V. Quinn
- Mr. Arman Sabbaghi
- Mr. Jonathan M. Schaab
- Mr. Brian T. Schwartz
- Ms. Rachel C. Smith
- Mr. Sebastian S. Smith
- Ms. Stephanie L. Spencer
- Mr. Kenneth Wakeland
Actuarial Science Majors (* indicates Actuarial Science Honors)
- Ms. Noor M. Al Sayed *
- Mr. Craig A. Allen *
- Mr. Steven M. Balog *
- Mr. Li Chen
- Mr. Andrew Chung
- Mr. Adam R. Crone *
- Ms. Kristen E. Dyson *
- Ms. Sarah M. Haynes
- Ms. Gita A. Himawan
- Mr. Michael D. Hoyer
- Mr. Dhaval M. Jadhav *
- Ms. Petrina Kusliawan *
- Ms. Lisa McGuire *
- Mr. Brett R. Newman
- Mr. Samuel V. Oglesby
- Ms. Kathryn R. Patterson
- Ms. Katherine V. Quinn
- Mr. Jonathan M. Schaab
- Mr. Brian T. Schwartz
- Ms. Rachel C. Smith *
- Mr. Sebastian S. Smith
- Ms. Stephanie L. Spencer
For the academic year 2008-2009, 10 Ph.D. students, 20 M.S. students, 7 Graduate Certificate students, 41 Undergraduate Statistics students and 28 Actuarial Science students received degrees from Purdue University and the Department of Statistics.
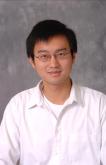
Zhongyin (John) Daye
We would like to highlight the achievements of one of the Department's May 2009 graduates. Dr. Zhongyin John Daye has carried out researches in the important area of model selection and estimation for high-dimensional regression. His research interest is directed towards the development of new methodologies for high-dimensional model selection that are intuitively appealing, computationally efficient, theoretically sound, and easily extendible to new situations.
In a published journal paper, Dr. Daye, with Dr. Xinge Jessie Jeng, also one of the Department's May 2009 graduates, proposes the weighted fusion, a new penalized regression method that utilizes correlation information from the data to improve variable selection under multicollinearity. The weighted fusion is motivated by applications, such as gene microarray analysis, where data often exhibit multicollinearity due to the presence of genes or variables that belong to the same system or pathway. Popular variable selection procedures, such as the Lasso (Tibshirani, 1996), tend to select only a few variables arbitrarily from a group of correlated variables. This can be disconcerting in many applications. For example, when studying a genetic disease, genes that might directly contribute to a malignant trait may not be selected in the presence of correlated variables. The weighted fusion penalizes pairwise differences of coefficients via correlation-driven weights to allow related variables to be selected together. Results show that weighted fusion can often outperform the lasso and elastic net (Zou and Hastie, 2005). It is also useful when the number of predictors p is larger than the number of observations n by allowing selection of more than n variables. The weighted fusion introduces a new approach for controlling multicollinearity in variable selection.
In other work, Dr. Daye, with collaborators, proposes the covariance-thresholded lasso, a new penalized regression method for variable selection in high-dimensions. It employs covariance regularization (Bickel and Levina, 2008) to stabilize and increase the rank of the sample covariance matrix for the lasso by utilizing possible sparsity of the underlying covariance matrix. Covariance sparsity, in turn, is a natural assumption and appears in many applications, such as gene microarray analysis and image processing, where a large number of predictors are independent or weakly correlated with each other. Results show that covariance-thresholded lasso can often outperform the lasso, adaptive lasso (Zou, 2006), and elastic net for variable selection when p is very large but n is small. The covariance-thresholded lasso makes an important contribution in attempting to answer the question: can sparsity of the covariance matrix be utilized to improve variable selection, and, if so, why?
Dr. Daye has accepted a two-year postdoctoral position at the University of Pennsylvania with this year's invited Myra Samuels Lecturer, Professor Hongzhe Li. He will work with Professor Li to develop new statistical methodologies for the high-dimensional genomic science.
Best wishes to all of our graduates. We look forward to hearing from you!