Congratulations 2008-2009 Graduates
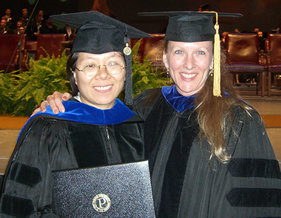
Dr. Lingling An performed her Ph.D. research in the area of high-dimensional dynamic cluster analysis under the direction of Professor Rebecca W. Doerge. Dr. An, an active member of the Statistical Bioinformatics Center, participated in a great number of very productive side projects during her time at Purdue University. In particular, Dr. An has worked closely with the members of the National Science Foundation funded "Functional Genomics of Plant Polyploids", as well as the National Institute of Health funded "Molecular Analysis of Synaptic Transmission Mutants". The title of Dr. An’s dissertation is: "Dynamic Clustering of Time Series Gene Expression".
Typically, when gene expression profiles are clustered for the purpose of understanding the functional co-regulation of genes, genes with similar profiles are organized into groups such that each gene can be a member of only one group or cluster. Early on, Lingling realized that genes participate in multiple biological processes and thus it is of interest to cluster genes in a manner that allows genes to belong to multiple clusters.
Visually, genes that participate in multiple clusters may have very different expression patterns. By taking advantage of techniques and theories from signal processing, Dr. An clustered periodic gene expression profiles from a dynamic perspective under the assumption that different biological processes are characterized by different spectral frequencies. Lingling proposed a dynamic clustering method which provides insight into the dynamic associations among time-limited co-expressed genes. The novel contributions of Dr. An’s work in the areas of Statistics and Bioinformatics are two-fold and include the concept of time-varying clusters, as well as an approach to differentiate significant, or biologically meaningful, clusters from noisy clusters.
After graduation, Dr. An relocated with her husband James, three children (Richard, Annie and David) and dog (Dobbie) to Tucson, Arizona where she will be an Assistant Professor in the Department of Agricultural and Biosystems Engineering, University of Arizona.
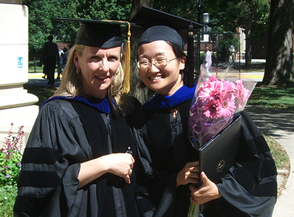
Dr. Suk-Young Yoo is the first Statistical Bioinformatics Center Ph.D. student to study the statistical issues surrounding the analysis of data from epigenomic experiments. The title of Dr. Yoo’s dissertation is: "Statistical Methods for Integrating Epigenomic Results".
Epigenetics is the study of heritable alternations in gene function without changing the DNA sequence. Epigenomics is the genome-wide study of the distribution of epigenetic modifications in the genome. It is known that epigenetic modifications such as DNA methylation and chromatin modifications are highly correlated with regulation of gene expression. Both DNA methylation and histone lysine methylation have been associated with human cancer and genome instability. Suk-Young’s research relied on a new type of microarray called a tiling microarray. Tiling array technology has played a large role in evaluating gene expression, methylation, and histone modifications for thousands of genes simultaneously across a whole genome. Since tiling arrays consist of DNA sequences, referred to as probes, their linear ordering provides a ‘tile path’ of evenly distributed overlapping, non-overlapping, or partially overlapping tiles that span a genomic region or even the whole genome.
To date very little work has been done to establish novel statistical methods that exploit the relationship between epigenetic modifications and gene expression. Dr. Yoo initiated a novel statistical approach to evaluate gene expression changes as related to DNA methylation and histone lysine methylation for the purpose of examining the effects of epigenetic modifications on gene regulation. This research explores the relationship between gene expression and epigenetic modifications using a two-stage analysis that employs Hidden Markov models and linear models to combine data and results from both methylation tiling and gene expression tiling arrays. The motivation for the two-stage approach comes from the process of assessing methylation changes of tiles where both biological and technical error can cause differences between the true methylation status and the observed methylation status, which in turn may lead incorrect results when estimating gene expression. By breaking the analysis into two stages, the estimation of methylation status per tile benefits from the Hidden Markov model application by utilizing information of neighboring tiles so that when the results of the methylation analysis are incorporated into the evaluation of differential expression, more precise estimation of gene expression (i.e., differential expression) can be obtained. The benefits of this two-stage approach are its flexibility and the fact that the same idea can be further extended to combine results from histone modification experiments (i.e., H3 lysine-4 dimethylation (H3mK4) and H3 lysine-9 dimethylation (H3mK9) using chromatin immunoprecipitation with microarray technology (ChIP-chip arrays) to evaluate gene expression of tiles given changes of methylation of histones or interactions between DNA methylation and histone methylation.
After graduation Dr. Yoo joined M.D. Anderson Cancer Center in Houston, Texas as a Research Statistical Analyst.
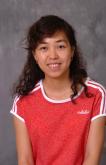
The title of Dr. Zeng’s dissertation is: "Group Variable Selection Methods and Their Applications in Analyses and Genomic Data."
Variable selection methods are powerful tools in analysis of high dimensional massive data. In bioinformatics, the methods have often been applied in gene expression microarray data to reduce dimensions and select important features. It is well known that for genes participating in a common biological pathway or sharing a similar function, the correlations among them can be very high. However, most of the available variable selection methods cannot deal with the complicated interdependence among data. We propose three new methods, via two different approaches, by selecting groups of variables in regression models. First, we propose two new selection algorithms, namely gLars and gRidge, following LARS forward selection procedure. The new approaches intend to conduct grouping and selecting at the same time, not requiring any prior information on group structures of the variables. The third method called SCAD-L2 is a penalized regression method. Lasso, a popular regularization approach, utilizes L1 penalty. Elastic net combines L1 and L2 penalties to incorporate group effects in the variables. However, both of them provide biased coefficient estimators. The biasedness of Lasso and elastic net interferes with variable selection. Fan and Li (2001) proposed a non-concave penalty function called SCAD with many good properties, including unbiasedness. Our new method SCAD-L2 combines the penalties of SCAD and L2. It favors group effects in addition to the good properties of SCAD. Simulations show that our proposed methods often outperform the existing variable selection methods, including Lasso, LARS, SCAD and elastic net, in terms of both reducing prediction error and preserving model sparsity, while yielding additional group information. We apply the proposed methods in gene expression microarray data and genetic variant SNP data. The group variable selection models are more appropriate than other existing methods for the genomic data with complicated interdependent structures.
After graduation Dr. Zeng took a position as a Biostatistician at MedImmune, a pharmaceutical company located in Gaithersburg, Maryland.