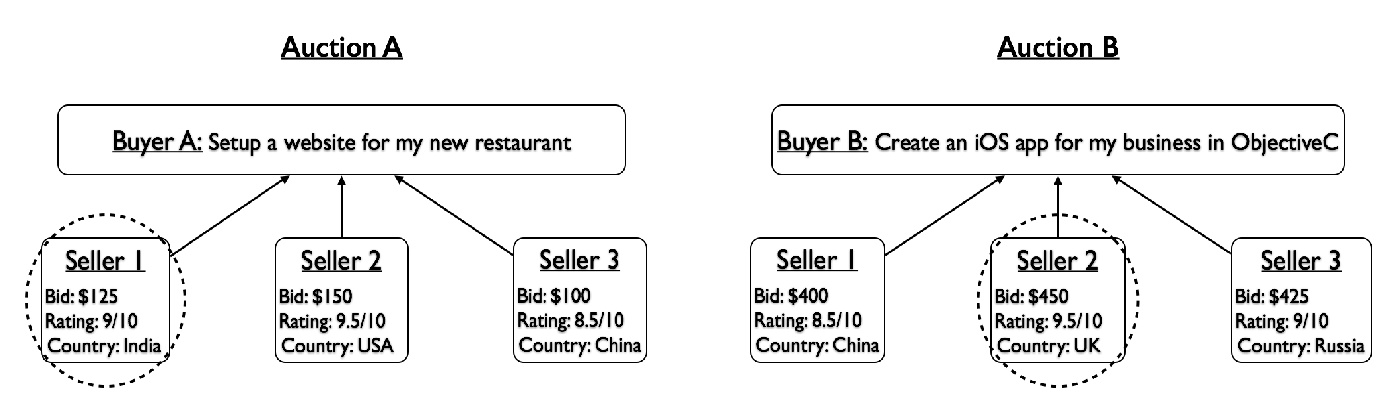
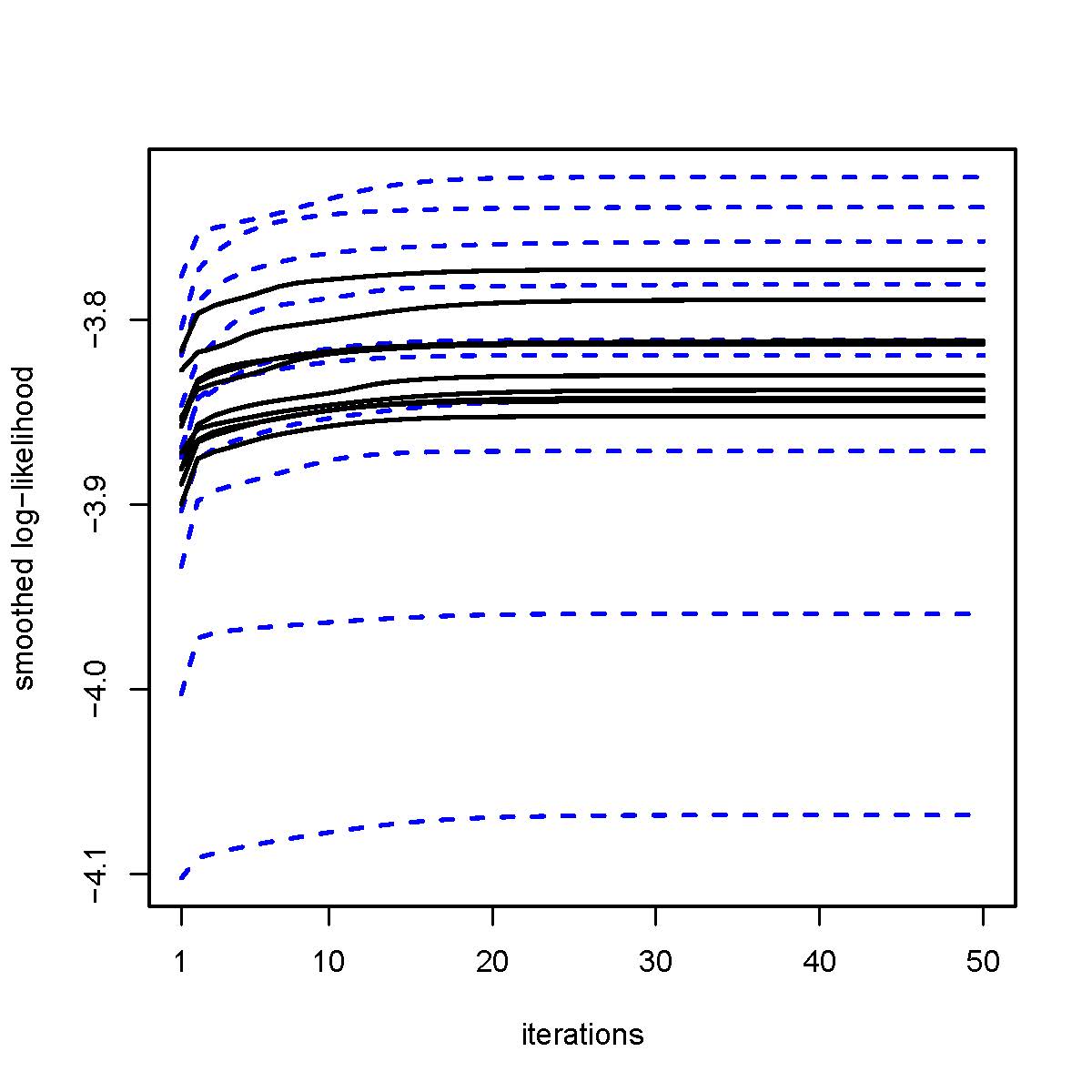
Non- and semi-parametric multivariate density mixtures
The multivariate non-and semiparametric density mixtures are widely applicable in many areas of statistics. They are particularly useful in clustering and classification of multivariate high-dimensional data. The majority of model-based clustering techniques for multivariate data is based on the multivariate normalmodels and their direct generalizations. However, this approach is quite restrictive since, in most cases, it is difficult to model clusters of the non-elliptical shape with it. If one is not restricted to multivariate elliptical distributions, this issue disappears; however, models of non-elliptical multivariate distributions are much harder to understand. Moreover, the choice of specific parametric form of components of such a model in practice is often rather arbitrary and motivated, for the most part, by considerations of technical convenience. Also, a commonly encountered and practically important case where one needs to cluster multivariate data of mixed type that include both continuous and discrete random variables is impossible to do parametrically. All of the above leads us to consider non- and semiparametric multivariate density mixtures in their full generality.
The simplest multivariate nonparametric density mixture model is the one that assumes conditional independence of marginal distributions. This model has a fairly wide range of applicability; however, the assumption of conditional independence is rather impractical in many areas of science. For example, if clustering jointly expressed genes using RNA-sequencing data, such an assumption implies independence of expression under various experimental conditions which is usually cannot be assumed.
To avoid this problem, we propose to model modeling of a vast variety of dependence structures for multivariate clustering and classification using various copulas. This approach also allows a conceptually straightforward way of clustering for datasets containing both continuous and discrete data without having to fit a very large number of parameters. For many models, this approach results in succinct and efficient algorithms that can be shown to converge with respect to an explicit objective function. Many of these algorithms are of the MM (Majorization-Minimization) type that is a generalized version of the EM (Expectation-Maximization) approach.
It is an interesting fact that estimation for some of these models can be thought of as Tikhonov type regularization problems. The study of these regularization problems is needed in order to understand the large sample behavior of solutions obtained by employing the algorithms just described. This study is also a part of this project. It requires the use of calculus of variations and theory of perturbations of linear operators.
One interesting application area of these algorithms is the economic game theory. There, they have been used to estimate components of the so-called beauty contest auctions. Beauty contest auctions are auctions where the buyer of goods/services does not have to disclose rules he/she follows in selecting auction winners. Such auctions are often held online when looking for freelancers.
Nonparametric estimation of variances and other moments
The function estimation is of profound importance in many applications. Originally, it developed from interest in signal estimation and transmission; today, other areas of application, such as image analysis, are of at least equal interest.
Our current research interest lies mostly in the area of autocovariance function estimation and inference, focusing mostly on the case of non-stationary (typically, locally stationary) time series. Such an estimation is needed for the purpose of optimal forecasting of the corresponding series, the efficient estimation of time series regression models and the inference of time series regression parameters. Our approach is often based on the use of the so-called difference sequence method whose origins lie in the early research on time series. The methods we propose are also applicable to nonparametric estimation of autocovariances in spatial models, including non-stationary ones.
Properties of multivariate truncated distributions
Our work in this area is motivated by the notable practical usefulness of multivariate truncated distributions (e.g. multivariate truncated normal, multivariate truncated elliptical etc.). Their theoretical understanding is, however, rather limited and this has inspired us to try to explore their properties in greater details. In one of our recent manuscripts we established, apparently for the first time, that multivariate truncated normal distributions belong to an exponential family that is neither steep nor regular. This implies certain difficulties concerning maximum likelihood estimation approach of its parameters. Multivariate truncated distributions are used in many areas of science, including simultaneous equations inference and multivariateregression, economics and econometrics, educational studies, and inference with censored biomedical data. This motivated our recent investigation of the maximum likelihood estimation of its parameters. Our future work in this area will be dedicated to more detailed investigation of this and related topics, such as the properties of more general multivariate truncated elliptical distributions.